EXL's Guide to fine-tuning LLM
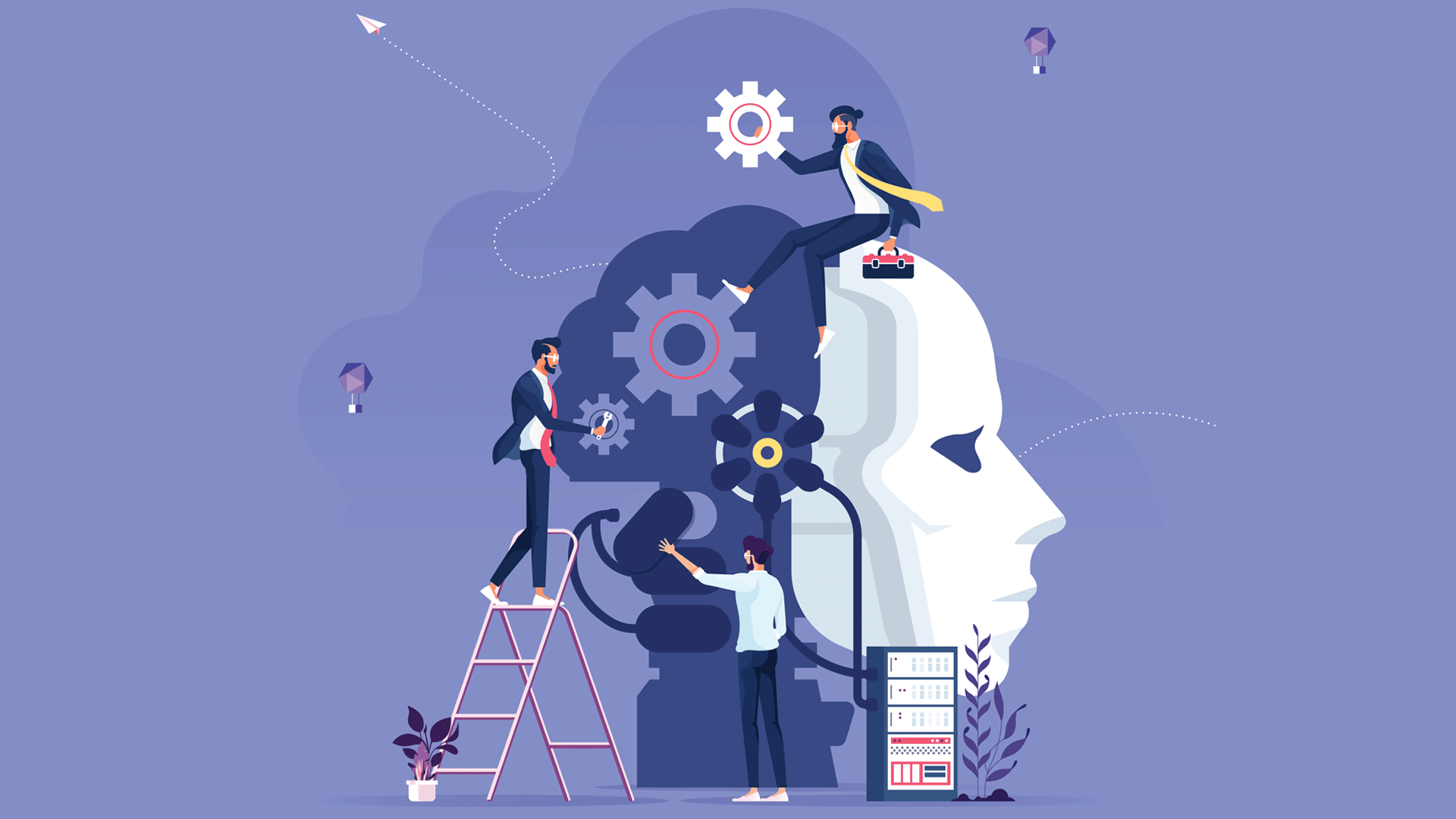
Traditional, off-the-shelf Large Language Models (LLMs) often fall short, lacking the fine-tuning needed for the nuanced challenges of faced by insurance companies during claim adjudication.
This has led to inefficiencies, high indemnity costs, claims leakage, longer settlement timelines, and increased compliance risks.
Recognising these challenges, EXL has leveraged decades of industry expertise to develop a solution that ensures accuracy, reduce cost, and improve consistency in industry-specific AI applications.
The company's internal studies show a remarkable 30% improvement in task accuracy compared to leading pre-trained models like OpenAI GPT4, Claude and Gemini.
But they are intrinsically limited to retrieving knowledge that is present in the training data.
While these foundational models are trained on publicly available data, teams will need to use private data to train LLMs if they want to create a high-performing and useful generative AI (GenAI) solution for their organisation.
So, while organisations race to apply GenAI to enhance products, customer experience, and operations, they face challenges in fine-tuning models designed and trained on publicly available information.
And yet, applying GenAI to complex, industry-specific use cases is how most organisations use or plan to use these models.
According to Gartner, “By 2027, more than 50% of the GenAI models that enterprises use will be specific to either an industry or business function.”
With a focus on real-world applications, this guide provides a clear pathway for deploying effective industry-specific AI solutions, empowering you to optimize your AI initiatives successfully.
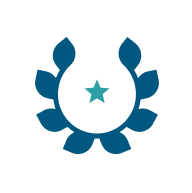
Comments
Remove Comment
Are you sure you want to delete your comment?
This cannot be undone.