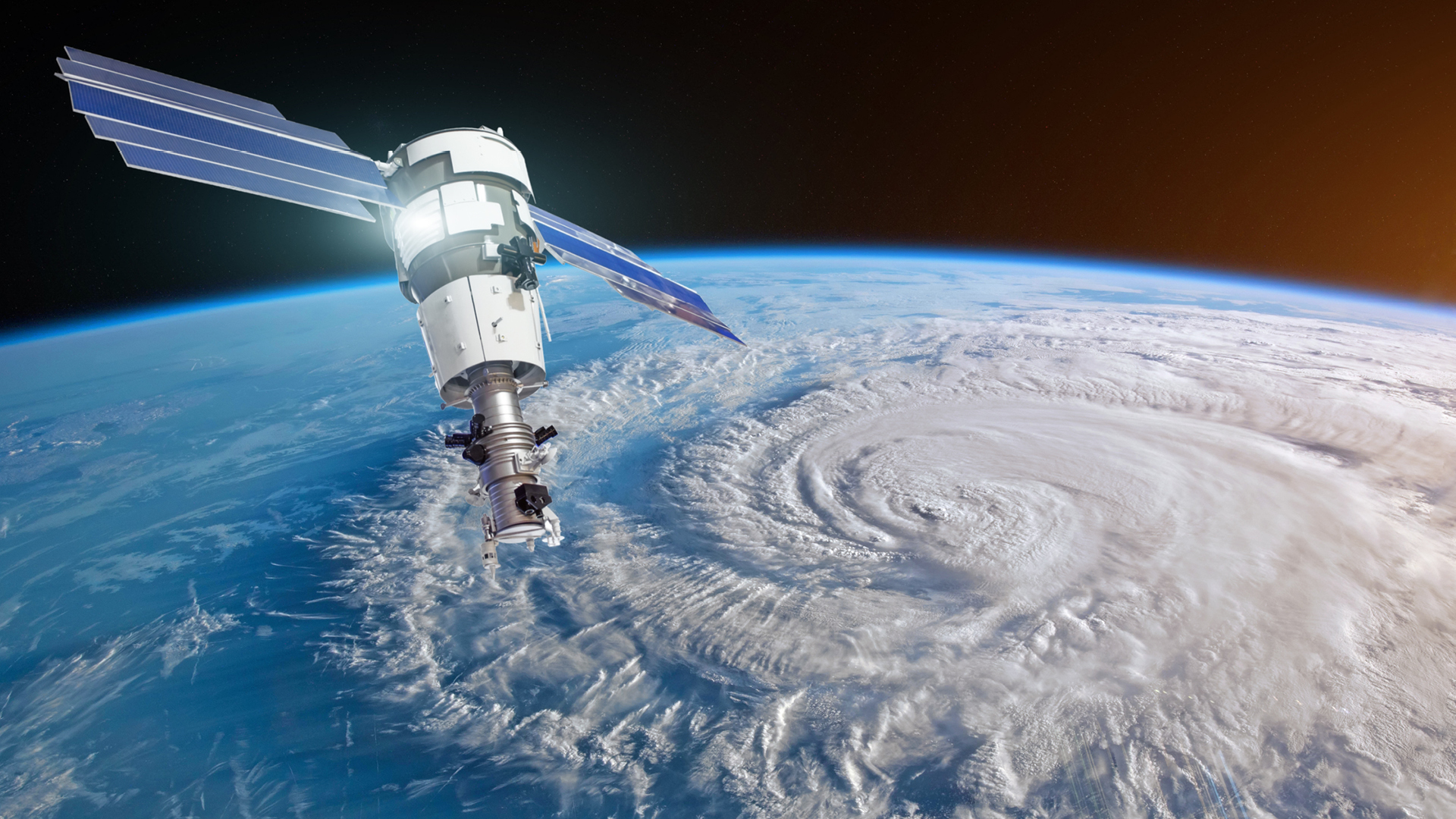
Vol 46: Issue 4 | December 2023
Today’s low-orbiting satellite technology is so ubiquitous and so precise that it can deliver information about roof types on buildings in particular geographic areas. The satellite images can discern flat roofs and pitched roofs, and can even help make an assessment of what condition they are in.
At data analytics and risk assessment firm Verisk, the information can be assessed through machine learning and artificial intelligence (AI) techniques that also combine up to 50 years of climate data, not only on temperature but also on hail, wind speed and barometric pressure.
“The result is catastrophe modelling that aggregates hundreds of thousands of data points and uses AI training algorithms to create a multitude of potential scenarios,” says Verisk’s executive vice president and managing director for the EMEA and APAC regions Dr Milan Simic.
“For reinsurers this means more insight than ever, as they price risk and consider their appetites and capacity.”
Simic says Verisk looks at what happened in the past but aims to create models that are fit for what the company calls the near-present climate.
“Insurance contracts are usually one year, although some will be out to two or three years, so we need to make sure that the models are appropriate for this near-present climate — but also have one eye on what is likely to happen in the future,” he explains.
“Obviously, we are in an environment of climate change, and we need to price risk not just for today but also the models need to be able to create what we call conditioned catalogues for the future so we can assess those potential losses.”
Past and future thinking
The world of catastrophe modelling has changed significantly from its inception in the late 1980s and early 1990s. It emerged as a response to hurricanes Elena (1985) and Andrew (1992) in Florida and a series of earthquakes in California which peaked at magnitude 7.3 in 1992. Under this pressure, several insurers went under, due to a lack of understanding of their potential losses.
Prior to these events, the reinsurance industry was modelling natural disasters using actuarial principles and the discipline of statistics. Fast-forward to today and sophisticated modelling is now fully embedded in the risk transfer chain.
While the models don’t claim to forecast what might happen in the future, they are built with the understanding that world weather patterns are changing and that historical data is not necessarily predictive.
“For Australia, we have several different models for earthquakes, cyclones, severe thunderstorms or hail and bushfires — and for New Zealand we also model for earthquakes,” says Simic.
Elsewhere in APAC, Verisk also has flood models for China, Japan and Thailand.
“So, we model hundreds of thousands of potential events and their impact, meaning the losses and the implications for reinsurance companies. For example, the models tell us about properties that are insured, and we put geolocation on those properties and that gives you potential outcomes of losses.”
This process delivers an exceedance probability curve, which gives the likelihood that losses will be more than a certain threshold, and these are then used in the risk transfer chain by both reinsurers and insurers to price their policies.
Data-hungry models
At risk management and professional services firm Aon, senior catastrophe research analyst Dr Tom Mortlock leads APAC’s climate risk advisory and is also adjunct fellow at the Climate Change Research Centre at the University of New South Wales.
Mortlock says that, while the methodology and structure of the catastrophe models have largely remained the same, the ability to analyse an exponentially bigger number of data points through machine learning has been the big game changer.
“Essentially, we are still using a bottom-up approach [collecting and aggregating data related to the weather, geography, vegetation and habitation], which has remained similar to how it was when catastrophe modelling began,” he says.
“There are pros and cons in that approach. Bottom-up means you can be very granular on what you produce at the portfolio level, but on the downside it also means that the models are very data hungry.”
The challenge of working with such high volumes of data, Mortlock explains, is managing the quality. If data isn’t properly assessed and cleaned, it can impact the forecasts and make the models less accurate.
The best results, he says, are when you combine the climate modelling with an understanding of where assets are and the nature of those assets. Using details such as floor heights in the modelling, for example, can make a significant difference in understanding potential losses.
While insurance contracts are typically priced for the next 12 months, this pricing is often undertaken using a long-term mean estimate. Nowadays, a concern is to ensure the ‘old climate’ is not being used in this mean estimate.
“We certainly don’t want to be building catastrophe modelling with any information which is much beyond the last few decades, because we are in a different climate state now,” says Mortlock.
“What catastrophe models are essentially trying to do is understand tail risk a little bit better, because that is the bit which insurers want to transfer to reinsurers. Observations alone often fall short in this regard, as they are just not long enough to properly capture the tail, and so for this we turn to cat models.”
This is where catastrophe modelling has evolved to. Where once it might have delivered scenarios on extreme weather events and their incidence, today’s technology is finding ways to better understand the impact on insured assets because the characteristics of those assets have become part of the modelling.
This might not be forecasting the future, but it is giving insurers a more accurate understanding of what is at stake, so they can price it and protect it accordingly.
Avoiding tunnel vision
Nick Hassam is a co-founder of Sydney-headquartered technology start-up Reask, which builds solutions for the reinsurance and insurance industries to manage their exposure to climate risk.
Hassam set up Reask five years ago with a colleague experienced in machine learning techniques.
They have built a new framework for catastrophe risk modelling that takes global climate models and analyses huge volumes of information, such as sea temperatures, sea-level air pressure and upper-level wind shear.
Taking 40 years of data divided up into monthly segments, Reask might be working with up to 200 different parameters. Using machine learning, the framework then converts these insights into algorithms that build views of risk which help to determine the frequency and severity of events.
It is also able to look at future climate scenarios under different scenarios, such as one or two degrees of warming, to create an understanding of future climate expectations.
Reask used two supercomputers in Australia, located in Canberra and in Perth, to build its machine learning training dataset.
“The volume of data is such that, with these volumes, we really need high-performance computing to push out these simulations,” says Hassam.
“Essentially, the machine learning reduces the insights in that massive volume of data down to something that is more manageable and consumable.”
The Reask business model is data-as-a-service. Most clients access pure data from Reask and then run their own risk and pricing models. They will also consult a range of sources and opinions, because as reinsurers they understand that there is a risk in confining decisions based on only one viewpoint.
“Clients will take our service and that of a competitor and an actuarial model and internal view, because regulators stipulate that (re)insurance companies need to take multiple views,” says Hassam.
“If you took just one view of risk, you would be building up a significant level of systemic risk.”
Fire risk and El Niño
As the world enters an El Niño weather pattern, experts are weighing in on what that means for bushfire risk.
In New Zealand, scientist Hugh Wallace from research institute Scion believes the hot, dry and gusty winds that typify El Niño conditions are likely to slightly increase the local bushfire risk. However, he and other experts are quick to point out that almost all New Zealand bushfires are started by people — a factor that cat models struggle to predict.
In Australia, a similar increased risk of bushfire is predicted, and fuel levels are higher than normal because of three cool, wet La Niña seasons in a row.
The Bureau of Meteorology introduced new modelling in 2022, called the fire behaviour index. It incorporates a more detailed view of vegetation density and types in specific areas, and how a fire will behave in different conditions.
Once again, what cat models can’t predict is ignition points (for example, from lightning strikes), but once a fire has started, they can predict fire intensity and direction much more accurately than older models could, drawing on real-time weather data, topography and vegetation information.
Open-source solutions
Whether we’re talking cyclones (in the Indian Ocean and south Pacific), typhoons (in the north-west Pacific) or hurricanes (in the north Atlantic), tropical storms can cause enormous destruction.
The University of California has launched a free, open-source catastrophe model to help governments in at-risk countries prepare better for storm risk and also work out disaster response plans.
The model combines tropical storm risk modelling with information on household vulnerability, so authorities can identify communities that may need more support if they are impacted by a storm.
“What catastrophe models are essentially trying to do is understand tail risk a little bit better, because that is the bit which insurers want to transfer to reinsurers.” says Dr Tom Mortlock from the Climate Change Research Centre.
“To understand that satellite images were so powerful that they could determine roof types in particular areas and factor that information into catastrophe modelling brought home to me how sophisticated cat modelling has become. It also made me realise that the reinsurance industry must be on the cusp of a major transformation driven by next-generation technology.”
Read this article and all the other articles from the latest issue of the Journal e-magazine.
Comments
Remove Comment
Are you sure you want to delete your comment?
This cannot be undone.