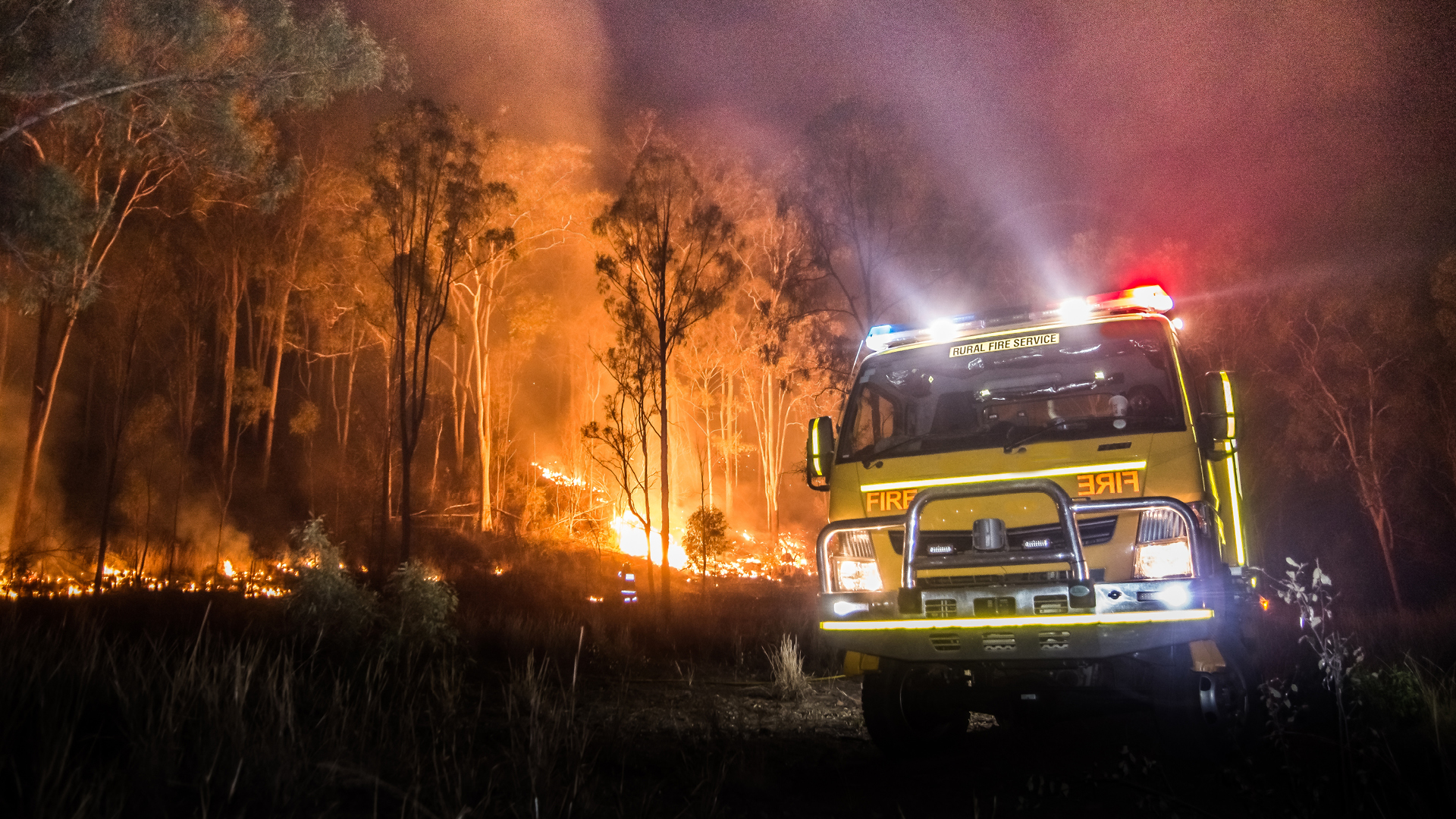
In short
- Technologies like artificial intelligence, machine learning and drones are improving the accuracy of bushfire risk modelling.
- This is helping reinsurers better understand and price their risks.
- But experts warn modelling alone won’t mitigate the risks. Others need to act on the data to develop better building and planning policies and codes, control fuel loads and tackle climate change.
Insurers can’t predict the next climate crisis, but we are getting close.’ That’s a tagline from Kettle Re, a San Francisco-based start-up reinsurer that uses artificial intelligence and machine learning (ML) to predict the likelihood of a wildfire in any given area.
Kettle Re’s catastrophe (cat) modelling software ingests data from 47 different sources, including NASA satellites, weather satellites and laser-based lidar mapping sensors.
Circumstances that make fires more likely, such as dry undergrowth, high winds, little rainfall and hot temperatures, are also picked up. For every analysis, models run 42 million simulations using a statistical approach called swarm neural networks.
By providing what it believes is a more accurate view of the fire risk, Kettle Re hopes to make the risk more understandable and more insurable.
So far, the expected loss ratio for 2020 wildfires for most major primary carriers that underwrite for wildfire damage is well above an unsustainable 100 per cent. However, Kettle Re says a simple and direct application of its risk estimate of wildfire probabilities improves pricing and reduces the loss ratio by significantly more than half.
Tech spreads like wildfire
Kettle Re’s choice of technologies for bushfire cat modelling appears to be where the reinsurance industry is headed.
According to Peter Cheesman, head of analytics for Asia Pacific at Aon, recently developed models use moderate resolution imaging spectroradiometer (MODIS) satellite data to better track burnt areas, and ML to validate them. MODIS is a key multispectral sensor that is flown aboard NASA’s Terra and Aqua satellites.
These satellites view the earth’s entire surface every one to two days at a moderate resolution.
‘ML is also being used to define burning probability and directional spread probability of fires from historical data,’ says Cheesman, adding that cat models are not tools for predicting what any single fire season might look like.
Instead, he says, ‘these tools are entirely focused towards estimating the potential financial loss for capital requirements such as reinsurance, and ML is one of the latest techniques that model vendors are using to enhance their modelling accuracy’.
Rade Musulin, a principal at Finity, notes that satellite data and the use of ML to analyse imagery has led to greater accuracy when it comes to identifying high-risk properties and pricing the risk.
Other financial services companies are using similar technology.
Dr Pierre Wiart, head of consulting and risk management solutions for CoreLogic Asia Pacific, says that since the Black Summer bushfires of 2019-20, his company has been experiencing more demand for information and the latest technology to improve understanding of bushfire risks and mitigation strategies.
In Australia, CoreLogic has developed a bushfire monitoring solution. ‘We’re able to extract satellite imagery on a weekly basis that surveys and monitors Australia’s geographic mass and identifies the areas subject to bushfires,’ says Wiart.
‘We compare this imagery to our comprehensive database and identify properties that have been directly impacted.
‘It forms an important part of our broader natural hazard risk mitigation offering, which allows us to monitor other natural hazards, including floods, cyclones, hailstorms and more. Without this service, banks and the financial industry would have limited visibility of how their mortgage portfolios and customers might be impacted by these events.’
Drones flying high
Drones are also being used to collect data on the fire risk of individual properties worldwide.
In the United States, for example, start-up Firemaps is using drone and satellite imagery to help defend Californian homes from wildfires.
The company creates 3D maps of properties and uses them to draw up a plan to make the property more ignition resistant. Firemaps also offers a database of qualified general contractors to carry out the work required to mitigate the risks it uncovers.
The company claims it can greatly reduce the risk of a home being destroyed by wildfire. After wildfire risk mitigation work has been done, Firemaps completes customers’ wildfire readiness assessments and will share this information with their insurers on request, so customers can maintain insurance coverage and lower their premiums.
Understanding vs mitigating fire risks
When it comes bushfire cat modelling, Musulin says it is important to distinguish between information and action. ‘Cat models help us understand bushfire risk, and pricing for it helps motivate loss reduction. But mitigating risks also requires parties other than insurers to act on that information, such as land-use policy, stronger building codes, controlling fuel loads, fire suppression and, of course, tackling climate change,’ he explains.
‘With greater availability of satellite data and better technology to analyse that data at a detailed level, we are able to build up a very granular view of the vegetation surrounding any given property. This captures a significant portion of the risk at a property level. The influence of climate drivers is more difficult to understand and predict.’
A challenge to model
Despite the uptake of new technologies to model risks, Cheesman says bushfire remains one of the most challenging perils to model, due to several factors that may affect the loss.
‘I believe we have achieved an acceptable level in defining bushfire hazard. However, in terms of loss estimation, fire propagation modelling and vulnerability calculation, we still need to extend our knowledge.’
Cheesman says the main challenges of bushfire modelling are developing realistic burnt extents of the events and defining the underlying fuel layers.
‘For example, if a fire ignites in a semi-urban area, how far can the fire go and burn properties due to the defined fuel layers? The other challenge is trying to accurately predict how any single fire develops and how the severity might cause subsequent damage.’
Musulin adds that catastrophic bushfires have many causal factors, including multi-year climate cycles, local daily weather conditions, fuel loads and human behaviour and intervention.
‘While we can understand and form assumptions relating to each individually, the most costly bushfires generally occur from the “perfect storm” of these factors. Capturing how they interact can be challenging,’ he says.
Factors to consider in fire modelling
So, what variables should be considered in the modelling?
‘Factors like ground slope, area of bushland nearby, area of grassland nearby, surrounding buildings, aspect of nearest bushland and so on,’ says Cheesman.
‘Historical data is also really important in order to verify models, including event rates and burnt extent.’
Musulin says Finity employs a range of granular data relating to the property when it considers its risk, including the type, location and area covered by surrounding vegetation, nearby road breaks, topographical information and dominant wind direction.
‘In calibrating our models, we consider historical losses,’ he says. ‘However, with building standards changing, population shifts, climate change and changing fire suppression techniques, information
about the past is becoming less relevant to the future.’
What we can learn
‘Other regions like the United States include some other factors such as firefighting capacity and smoke damage in their models, which Australia perhaps needs to consider,’ says Cheesman. ‘Models in the EU are not as sophisticated as those in Australia and the US.’
Musulin adds: ‘Australia has very advanced cat modelling for bushfire risk, perhaps reflecting that it is a relatively larger part of our catastrophe loss profile than is the case in the US, where earthquakes and hurricanes are more dominant. Our modelling capabilities support a strong private insurance market. accurately predict how any single fire develops and how the severity might cause subsequent damage.’
Musulin adds that catastrophic bushfires have many causal factors, including multi-year climate cycles, local daily weather conditions, fuel loads and human behaviour and intervention.
‘While we can understand and form assumptions relating to each individually, the most costly bushfires generally occur from the “perfect storm” of these factors. Capturing how they interact can be challenging,’ he says.
AI scores one for reinsurers
In the United States, reinsurer Aon has formed a strategic partnership with insurtech Zesty.ai to make more detailed property data accessible to property and casualty insurers underwriting wildfire risk.
Zesty.ai has developed the Z-FIRE predictive model, which uses artificial intelligence and machine learning to score individual properties out of 10, in terms of how likely they are to be destroyed by wildfire. The model draws on high-resolution imagery, topography, building records, historical wildfire loss data and weather data.
Risk factors include when the property was built and the building materials used, roof shape, the type and density of surrounding vegetation, and how close a property is to areas considered at high risk of wildfire.
The scores can be used by underwriters to price risk more accurately — and to take a single-property view, rather than accepting or refusing cover based on a customer’s suburb or postal code. Z-FIRE scores can also help identify ways homeowners can mitigate wildfire risk through clearing and home renovation.
Insurers such as Farmers expect to write more than 30,000 new property insurance policies in California, using Z-FIRE to identify eligible customers who might previously have been considered too high risk for cover.
Comments
Remove Comment
Are you sure you want to delete your comment?
This cannot be undone.